In addition to their use as survey sample sources, voter files are commonly used in political research by matching and linking them to people who have responded to polls or are found in various lists such as members of the clergy or physicians. Efforts to link public vote records to surveys go back several decades prior to the existence of modern commercial voter files. In the 1980s, the American National Election Study attempted to link voter turnout records to its respondents by having interviewers visit local election offices where the respondents lived. This labor-intensive and expensive effort was later abandoned but has been revived with the availability of better quality digital files in individual states and the commercial files covering the entire country. The Cooperative Congressional Election Study is another prominent election survey that has matched respondents to a voter file.
The process of linking commercial voter file records to survey respondents (or any list, for that matter) might seem straightforward: Use the respondent’s name, address and other information to identify a voter file record for the same person. However, the matching process can falter if there are major differences in names (e.g., a maiden name vs. current married name), or addresses (e.g., if respondents have recently moved). Quirks in the data can also affect the matching process. And some individuals are simply not present in the commercial voter files at all. For uniformity, we accepted the data as the vendors sent it, knowing that for a variety of reasons (including those listed above), some vendors matched panelists that others did not.

To better understand and evaluate both the matching process and the properties of voter files, Pew Research Center attempted to match members of the American Trends Panel, its nationally representative survey panel, to five different commercial voter files. To be sure, there are more than five vendors that maintain comprehensive national voter lists, but the vendors used in this study represent five of the most prominent and commonly used voter files. Two of the files are from vendors that are traditionally nonpartisan, and three are from vendors that work primarily with clients on one side of the partisan spectrum – two that work with Democratic and politically progressive clients and one who works with Republican and politically conservative clients.4
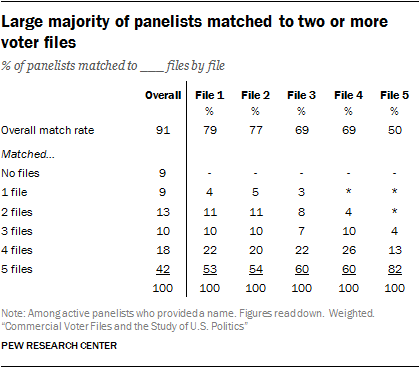
All vendors were provided with the same panelist information: name, address, gender, phone number, race and ethnicity, date of birth and email address. They were asked to find these individuals in their voter files using their normal matching methodology and return the voter file records, such as registration status and turnout history, to Pew Research Center. Of the 3,985 active members5 of the ATP who provided a name6, 91% were identified in at least one of the five commercial voter files. Vendors agreed to delete personally identifying information about panelists when the matching was completed.
When considered in total there is a high level of coverage of the survey panelists. But individual vendors matched at different rates. Two of the files (Files 1 and 2) matched the highest share of panelists (79% and 77% respectively) followed by Files 3 and 4 at 69% each.
File 5 matched at the lowest rate of just 50% of panelists. However, a low match rate does not necessarily imply lower quality data. In a follow-up analysis conducted to evaluate the quality of the matches, 99% of File 5’s matches were judged as likely accurate, compared with 94% to 97% of the other vendors’ matches. Voter file vendors told us that they have differing thresholds for confidence in selecting a match. This offers clients a trade-off in getting more data with more matches, at the cost of potentially including some inaccurate matches, versus fewer matches and greater accuracy but potentially more bias in the cases that are matched.7
Officials at File 5 said they were confident about the quality of their matches, which was borne out by our evaluation. However, they matched far fewer panelists than some other vendors and thus provided much less usable information overall, even if matches are limited to those who meet a high threshold of accuracy.
There is significant overlap in who matched to each file. Records for four-in-ten panelists (41%) were found on all five voter files and another 18% were found by four of the five vendors. Overall, 9% of panelists were found only on a single file – with most in this group coming from Files 1, 2 and 3). But each of the vendors found panelists that other vendors missed. Only 9% of panelists were not found by any of the vendors.
Matches made by File 5 (with the lowest overall match rate) have the highest overlap with other vendor matches. Fully 82% of matches to File 5 were also found by the four other files, followed closely by Files 3 and 4, with 60% of their matches being common matches with other files. Files 1 and 2 both had roughly half (53% and 54% respectively) of their matches found by all other files, with many of their matches found by only two or three other vendors.
How matches are made
The matching process uses information such as the respondent’s name, address, gender and date of birth – whether from a list or collected during the survey – to identify the respondent’s voter file record. Sometimes this process is straightforward, when a respondent’s name, address and date of birth match perfectly to the voter file. Unfortunately, this isn’t always the case. If the state voter list doesn’t report birthdays, or if a respondent is registered under a different name or at a different address, a successful match may not occur.
When a perfect match can’t be found, multiple possible matches must be considered, and the best match is chosen from among these. The process used by many vendors typically consists of two steps. The first step searches vast numbers of records to find potential matches, while the second chooses which among the plausible matches is best. At the first stage, the vendor’s matching software tries to identify all of the records that might be good matches to the respondent. Because the software has to sift through hundreds of millions of voter file records to identify these matches, computational shortcuts are used to locate plausible matches without burdening the software with assessing exactly which record will be the best match.
To give a concrete example, suppose that, at the time of this data collection, Vice President Joe Biden had been a part of our study. We would have asked the vendor to find the voter file record of a Joseph Biden, who reported being born in 1942 and residing (at the time) at 1 Observatory Circle, Washington, D.C., the official vice presidential residence. The software would set out to find all of the voter file records that could possibly refer to our respondent. People named Joe Biden or Joseph Biden, or having similar names like Jose Biden or Joe Widen, other 1 Observatory Circle residents and Bidens born in 1942 would all arise as possible matches. Once the full set of possible matches is generated by the first stage, the second stage begins. The software assigns all of the possible matches a score expressing the voter file record’s similarity to the respondent’s matching data. An exact name match would be assigned a higher score than approximate name matches like Jose Biden or Joe Widen. Similarly, matches that share a full birthdate or address would be assigned higher scores, while matches that merely live in the same city or that are the same age but have incomplete or nonmatching birthdates would receive lower scores. After all of these matching scores are generated, a best match is chosen.
Typically, the best match is simply the voter file record that mostly matches the information volunteered by the respondent. But other considerations can lead researchers to prefer a more imperfect match. Suppose we were left to choose between two records: a registered voter, Joseph Biden, with a listed home address in Wilmington, Delaware or a Joseph Biden, living at 1 Observatory Circle in Washington, D.C. but with no record of being registered to vote at that address. The Washington record is obviously the closer match, as it matches the address the respondent gave. On the other hand, if both records refer to the same Joseph Biden, then we may be more interested in the Delaware record, as the registered voter record will include information about his registration status, length of registration, vote history and political party. Ascertaining which of these two matches is preferred is partly a matter of making a trade-off between match confidence (the confidence we have that the record refers to the respondent) and the match’s usefulness (the amount of useful and relevant data conveyed by the voter file record).
When researchers have to match survey data to the voter file, they face the choice of doing the matching themselves. They can either take the whole voter file (or large portions of it) and write computer code to find the records that best correspond to the survey respondent, or they can opt to have a voter file vendor do it for them. Having a vendor do the matching is appealing, since it requires less work from the researcher and it can even be less expensive, since it means purchasing less data from a voter file vendor, but it comes at the cost of having less control over the matching process. When contracting out the matching process to a vendor, researchers typically never see the rejected matches, making it difficult to assess whether better matches were erroneously rejected by the vendor.
On the other hand, vendors have more experience matching and can usually devote more computational and software engineering resources to the problem than researchers can. Even if the methods are proprietary and not especially transparent, they could be a preferable option if their performance is superior.
Biases in the match
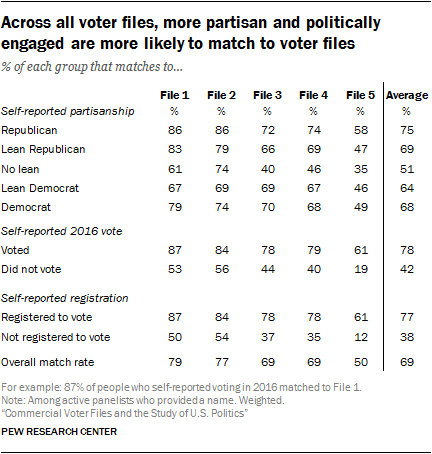
Failures to match do not occur randomly. Rather, certain kinds of people are less likely to be successfully matched. These individuals also tend to be different politically than those who are easier to match. This can lead to biases in conclusions drawn from data with matched voter file information. Panelists who are registered to vote and say they participate regularly in elections are more likely to be matched, leaving the politically disengaged underrepresented in voter files. This is to be expected, as registered voter lists in the states make up the bedrock of voter files.
In particular, Files 1 and 2 match more of those who are not politically active and engaged than the other vendors. Just 19% of those who said they didn’t vote in 2016 were matched to File 5. By comparison, 56% of 2016 nonvoters matched to File 2 and 53% were matched by File 1. A similar pattern emerges with voter registration. While File 2 matches 54% of those who say they’re not registered to vote, File 4 matches only about one-third (35%) of that group to their file, and File 5 – with the lowest overall match rate – matched only 12%.
A similar pattern appears with respect to party affiliation. Files with higher match rates, such as File 1, were able to match eight-in-ten or more of those who identify with a party to their file (86% of Republicans and 79% of Democrats), while 61% of those who do not lean toward either party were matched. While Republicans have a slightly higher match rate in several files, the partisan differences are modest.
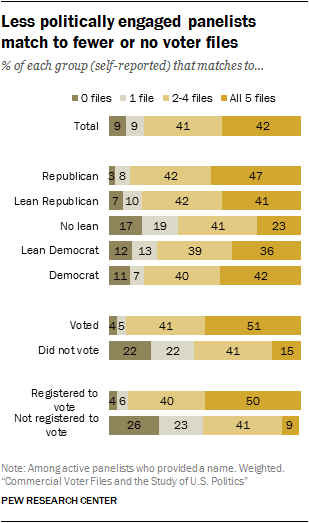
Differences in the match rates for different subgroups naturally have an impact on the demographic and political composition of those matched. While all files have a political engagement bias in terms of who is matched, those biases increase as match rates decrease. In other words, as vendors become stricter in terms of who they consider a match, the sample of people who are matched looks increasingly politically engaged. For example, 75% of American Trends Panel members say they voted in the 2016 election. Among those matched to File 1, the file with the highest match rate, 83% report having voted in 2016. Among those matched to File 5, the file with the lowest match rate, 90% report having voted.
Interestingly, these differences are not present when it comes to partisanship. While the partisan composition of the panelists matched to each of the five files is slightly more Republican than the panel overall, differences among the files are minor or negligible.
A consequence of these differences in match rates by partisanship and political engagement is that panelists who are registered to vote and regularly participate in elections are more likely to be matched to multiple files, while those who do not participate tend to be found on fewer (or no) files. Nearly two-in-ten who self-report not leaning toward either party (17%) are not able to be matched to any of the five voter files compared with just 3% of those who identify as Republican. Democrats and independents who lean Democratic are also slightly less likely to match: 11% of Democrats and 12% of Democratic leaners were not matched to any files.
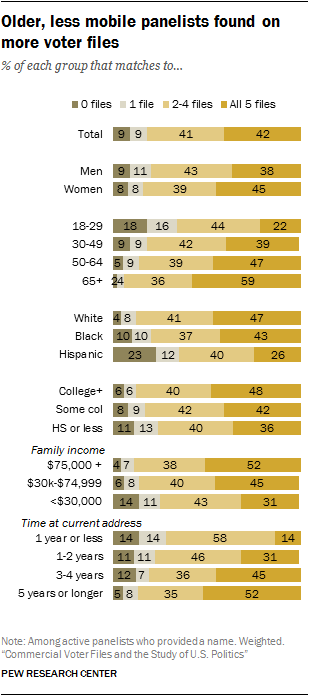
By the same token, those who identify with either of the parties are far more likely to be found in many, if not all, of the voter files in this study – a reasonable proxy for being easy to find. While just 23% of those who do not lean toward either party were found in all five files, more than four-in-ten Republican identifiers (47%) and Democratic identifiers (42%) were found on all five files. Those who lean toward either party, regardless of partisanship, were a little less likely to match across the files: Only 41% of Republican leaners and 36% of Democratic leaners matched to all five files.
An even more dramatic pattern can be seen with political participation. Half (51%) of those who reported voting in the 2016 election matched to all five voter files, compared with just 15% of those who said they did not vote. More than four-in-ten (44%) of those who said they didn’t vote were found in just one or no voter files, vs. 9% of those who said they voted. Among panelists who report not being registered to vote, 26% are not found on any voter files and another 23% match to only one file. Just 9% match to all five voter files.
Beyond the impact of political engagement, certain demographic characteristics are strongly associated with propensity to match. Voter files tend to do a better job of matching older, white, less mobile panelists while younger, more diverse and more mobile panelists end up with fewer or no matches. And, of course, these demographic characteristics are related to both partisanship and likelihood of participating in politics.
Age and mobility are particularly strongly associated with matching. Across all of the vendors, there is a roughly 30-point difference in the rate of matches between people ages 18-29 and those 65 and older. Similarly, people who have lived at their current address for less than one year are considerably less likely to be matched than those who have resided at their address for at least five years.
As a consequence of these patterns, the demographic profile of those matched differs somewhat across the vendors. File 5, with the lowest match rate, has the smallest share of panelists ages 18-29 (13% vs. at least 16% for the other files). And two-thirds of File 5’s panelists have lived at their current residence for at least five years, compared with 58% to 59% for the other vendors.
The demographic differences in propensity to match also mean that more than one-in-six younger panelists (18% of those ages 18-29) are not matched to any of the five files and an additional 16% were found on just one file. Only 22% of younger panelists were found in all five files. By comparison, 59% of older panelists (ages 65 and older) were found on all five files, and just 2% were not found on any of the files. Similarly, 52% of those who have lived at their current address for five or more years matched to all five files and just 5% could not be located in any file. Just 14% of those with less than one-year tenure at their current address were located by all five files.
Hispanics match at lower rates than other racial or ethnic groups. Nearly a quarter (23%) are not matched to any files. Only 26% of Hispanics were matched by all five files, while nearly half (47%) of whites were found by all five. Blacks fall somewhere in between. Roughly four-in-ten blacks (43%) were found on all five files, while 10% were not matched to any files.
While there are differences in propensity to match by educational attainment, they are comparatively minor. Half (48%) of panelists who report having at least a bachelor’s degree were matched to all five files, compared with 36% of those who reported having a high school diploma or less. Panelists at all education levels are roughly equally likely to not be matched to any file.