The analysis of Twitter users in this report is based on a nationally representative survey conducted from Nov. 21 to Dec. 17, 2018, among a sample of 2,791 U.S. adults ages 18 years and older who have a Twitter account and agreed to allow researchers to follow and report on that account. This study examines only the subset of respondents (N=2,427) who had public-facing accounts; private accounts are excluded. The margin of error for the full sample is plus or minus 3.3 percentage points.
The survey was conducted by Ipsos in English using KnowledgePanel, its nationally representative online research panel. KnowledgePanel members are recruited through probability sampling methods and include those with internet access and those who did not have internet access at the time of their recruitment (KnowledgePanel provides internet access for those who do not have it, and if needed, a device to access the internet when they join the panel). A combination of random-digit dialing (RDD) and address-based sampling (ABS) methodologies have been used to recruit panel members (in 2009 KnowledgePanel switched its sampling methodology for recruiting members from RDD to ABS). KnowledgePanel continually recruits new panel members throughout the year to offset panel attrition.
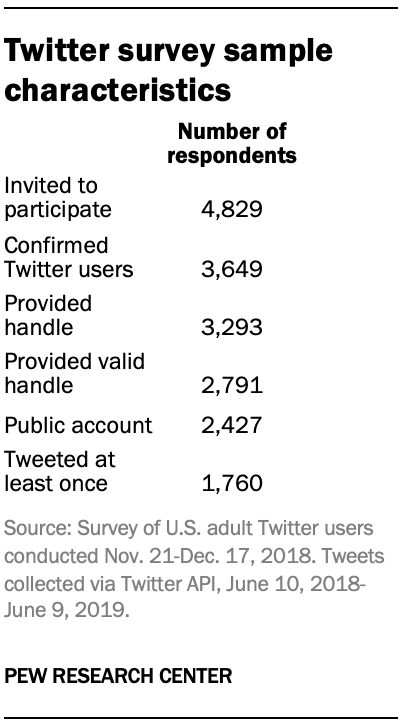
All active members of the Ipsos panel with an active Twitter account were eligible for inclusion in this study. In all, 4,829 panelists responded to the survey. Of that group, 3,649 (76%) confirmed that they used Twitter. Among confirmed Twitter users, 3,293 (90%) agreed to provide their Twitter username and complete the survey. Next, researchers reviewed each account and removed any that were nonexistent or belonged to institutions, products or international entities. Among the remaining 2,791 respondents who both completed the survey and provided a valid username (76% of confirmed Twitter users), 87% had public accounts. Of that set, 73% tweeted at least once during the time period of interest, a total of 1,760 individuals.
The complete sample of 2,791 adults was weighted using an iterative technique that matches gender, age, race, Hispanic origin, education, region, party identification, volunteerism, voter registration, and metropolitan area to the parameters of the American Trends Panel November 2018 survey of Twitter users. This weight is multiplied by an initial sampling, or base weight, that corrects for differences in the probability of selection of various segments of Ipsos’s sample as well as by a panel weight that adjusts for any biases due to nonresponse and noncoverage at the panel recruitment stage using all of the attributes described above.
Sampling errors and statistical tests of significance take into account the effect of weighting at each of these stages. In addition to sampling error, question wording and practical difficulties in conducting surveys can introduce error or bias into the findings of opinion polls.
Determining whether tweets mention national politics
Researchers started by choosing to collect tweets for one year surrounding the 2018 U.S. midterm elections (Nov. 6, 2018) and the dates of survey administration (Nov. 21-Dec. 17, 2018). All tweets posted by respondents with public accounts between 12 a.m. on June 10, 2018, and 11:59 p.m. on June 9, 2019, were included in the analysis, for a total of 1,147,969 individual tweets.
Next, researchers developed a definition of the concept of interest: national politics. Through an iterative process, researchers arrived at the following classification instructions: Political tweets are “defined as tweets that mention, or express support or opposition toward: national politicians, political parties, ideological groups, or political institutions, as well as mentioning formal political behavior.”
Researchers used a custom support vector machine text classification model – similar to the one used to classify news articles as mentioning different topics in this report – to label tweets as political or not. The model began with a set of keywords, hashtags and word stems related to national politics. That list included: realdonaldtrump, barackobama, mcconnell, obama, trump, president, republican, gop, democrat, congress, senat, politi, government, campaign, elect, vote, voting, bluewave, bluetsunami, #maga, #resist, resistance, conservative, liberal, conservative, and bipartisan. Researchers used regular expressions (a method of text searching) to avoid keyword false positives (such as catching words like “#magazine” when searching the term “#maga”).
Researchers also developed a list of keywords that were likely to occur in false positives (tweets that might appear to be about national politics, but that did not actually mention the topic). These terms included words like celeb, entertainment, food, gossip, leisure, movie and music, among others.
When training the classification model, researchers removed all tweets that contained both matches to the list of political keywords and matches to the list of false positive keywords. Researchers applied sampling weights to correct for changes in the sample’s representativeness that occurred as a result of this decision. Tweets that included words from the false positive list were included in the sample when making predictions and when evaluating the accuracy of the model.
Researchers estimated the model for an initial set of likely positive cases. Next, they applied a rule to override the model’s predictions based on the Twitter usernames (official, personal or campaign) of members of Congress, automated account usernames (which individuals can use to tag members of Congress), as well as the usernames belonging to President Donald Trump and former President Barack Obama. Any tweet that mentioned the username of a member of Congress, Trump or Obama met the definition of the concept described above and was automatically classified as a positive case.
Finally, four researchers coded the same set of 1,000 tweets such that each tweet was coded twice by different individuals. Researchers calculated the Cohen’s Kappa for agreement between the human coders. The Cohen’s Kappa for inter-rater reliability ranged from 0.82 to 0.88 across the pairwise comparisons. Researchers also calculated inter-rater reliability with respect to the model predictions. The Cohen’s Kappa for these pairwise comparisons ranged from 0.80 to 0.83.
Next, researchers combined the human codes in order to generate a list of 1,000 tweets that had been classified by two separate human coders (though not always the same two). In comparisons with the model’s predictions, the first set of human codes had a Kappa of 0.81 and the second had a Kappa of 0.82.
The model avoided false negatives and consistently identified true positives. Using the first set of human codes, the model’s precision was 0.89 and recall was 0.81. Using the second set of codes, precision was 0.87 and recall was 0.84.
Twitter data collection and prolific tweeters
The Twitter API limits how many tweets researchers can collect from individual accounts. Across the sample of users included in this study, the research team could not collect a complete timeline of tweets created between June 10, 2018, and June 9, 2019 for 2% of the sample (a total of 44 accounts). Even when excluding these accounts from the analysis, the results remain consistent.