Every election year, questions arise about how polling techniques and practices might skew poll results one way or the other. In the final weeks before this year’s election, the practice of “oversampling” and its possible effect on presidential polls is in the media spotlight.
Oversampling is the practice of selecting respondents so that some groups make up a larger share of the survey sample than they do in the population. Oversampling small groups can be difficult and costly, but it allows polls to shed light on groups that would otherwise be too small to report on.
This might sound like it would make the survey unrepresentative, but pollsters correct this through weighting. With weighting, groups that were oversampled are brought back in line with their actual share of the population – removing the potential for bias.
When people think about opinion polls, they might envision taking a random sample of all adults in the U.S. where everybody has the same chance of being selected. When selected this way, the sample on average will look just like the full population in terms of the share that belongs to different groups.
For example, the percentage of men and women or the share of younger and older people should fall close to their true share of the population. For the telephone surveys that Pew Research Center conducts, the process is a little more complicated (in order to account for things like cellphones and the fact that not everyone responds to surveys), but usually we want all adults to have an equal chance of being selected into the sample.
This works very well if you are interested in the overall population, but often we want to know what different kinds of people think about issues and how they compare with one another. When we are interested in learning about groups that make up only a small share of the population, the usual approach can leave us with too few people in each group to produce reliable estimates. When we want to look closely at small groups, we have to design the sample differently so that we have enough respondents in each group to analyze. We do this by giving members of the small group a higher chance of being selected than everybody else.
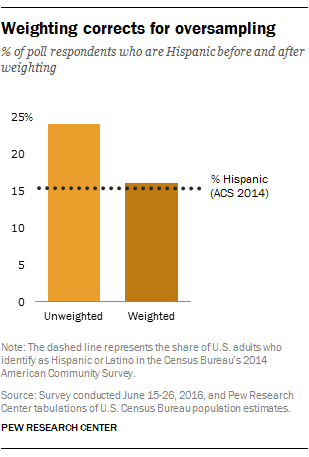
A good example is a Pew Research Center survey from June of this year, in which we wanted to focus in depth on the U.S. Hispanic population. In the previous survey from March, there were 291 Hispanic respondents out of 2,254 total respondents, or 13% of the sample before weighting. This is pretty close to the true Hispanic share of the population (15%), but we wanted to have more than 291 people responding so we could do a more in-depth analysis. In order to have a larger sample of Hispanics in June, we surveyed 543 Hispanics out of 2,245 total respondents, or 24% of the unweighted sample. This gave us a much larger sample to analyze, and made the estimates for Hispanics more precise.
If we just stopped here, estimates for the total population would overrepresent Hispanics. Instead, we weight them back down so that when we look at the whole sample, the share of Hispanics falls back in line with their actual share of the population. This way, we still have more precise estimates when looking at Hispanics specifically, but we also have the correct distribution when looking at the sample as a whole.
Pew Research Center’s 2014 Religious Landscape Survey also used oversampling in states like Wyoming so that researchers could make reliable estimates about Wyomingites’ religious beliefs and practices. Thanks to oversampling, we interviewed 316 Wyoming residents, instead of an estimated 63 under a non-oversampling design. The survey weighting adjusted for this by aligning the 0.9% of respondents from Wyoming with their actual share of share of the U.S. population (0.2%).