By Courtney Kennedy, Andrew Mercer, Scott Keeter, Nick Hatley, Kyley McGeeney and Alejandra Gimenez
As the costs and nonresponse rates of traditional, probability-based surveys seem to grow each year, the advantages of online surveys are obvious – they are fast and cheap, and the technology is pervasive. There is, however, one fundamental problem: There is no comprehensive sampling frame for the internet, no way to draw a national sample for which virtually everyone has a chance of being selected.
The absence of such a frame has led to lingering concerns about whether the fraction of the population covered by nonprobability approaches can be made to look representative of the entire population. For roughly 15 years, independent studies suggested that the answer to that question was generally “no” if the goal was to make accurate population estimates.1 Over time, though, researchers and sample vendors have developed technologies and statistical techniques aimed at improving the representativeness of online nonprobability surveys. Several recent case studies suggest a future (some would argue a present) in which researchers need not have an expensive, probability-based sample to make accurate population estimates.2
Key elements of the study
Design: 9 online nonprobability samples Comparison with an RDD-recruited panel 56 measures including 20 benchmarks
Analysis: Estimated bias on full sample results Estimated bias on subgroup results Estimated accuracy of regression models Demographic profile by sample Political profile by sample Variability of estimates across samples
To better understand the current landscape of commercially available online nonprobability samples, Pew Research Center conducted a study in which an identical 56-item questionnaire was administered to nine samples supplied by eight different vendors.
Nearly all of the questions (52) were also asked on waves of the Center’s probability-based American Trends Panel (ATP), which is conducted predominantly online but features mail response for adults who do not have internet access. The samples were evaluated using a range of metrics, including estimated bias on 20 full sample survey estimates for which high quality government benchmarks are available, estimated bias for major demographic subgroup estimates, and predictive accuracy of four different regression models. Among the most important findings of this study are the following:
- Online nonprobability surveys are not monolithic. The study finds, as a starting point, that the methods used to create online nonprobability samples are highly variable. The vendors differ substantially in how they recruit participants, select samples and field surveys. They also differ in whether and how they weight their data. These design differences appear to manifest in the samples’ rankings on various data quality metrics. In general, samples with more elaborate sampling and weighting procedures and longer field periods produced more accurate results. That said, our data come from just nine samples, so the effects of these factors are not well isolated, making these particular conclusions preliminary at best.
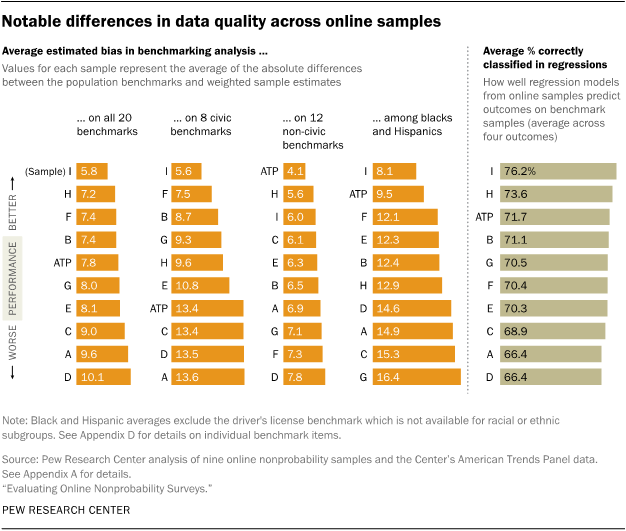
- Some biases are consistent across online samples, others are not. All the samples evaluated include more politically and civically engaged individuals than benchmark sources indicate should be present. The biases on measures of volunteering and community problem-solving were very large, while those on political engagement were more modest. Despite concerns about measurement error on these items, it is accepted that these errors are real because several studies have documented a link between cooperation with surveys and willingness to engage in volunteer activities.3
There is also evidence, though less consistent, that online nonprobability samples tilt more toward certain lifestyles. Most of the samples have disproportionately high shares of adults who do not have children, live alone, collect unemployment benefits and are low-income. In some respects, this squares with a stereotype one might imagine for people who find time to participate in online survey panels, perhaps akin to a part-time job. On other dimensions, however, the online nonprobabilty estimates are either quite accurate (e.g., have a driver’s license or length of time at current residence) or the biases are not in a consistent direction across the samples (e.g., daily smoking).
- Widespread errors found for estimates based on blacks and Hispanics. Online nonprobability survey vendors want to provide samples that are representative of the diversity of the U.S. population, but one important question is whether the panelists who are members of racial and ethnic minority groups are representative of these groups more broadly. This study suggests they are not. Across the nine nonprobability samples, the average estimated bias on benchmarked items was more than 10 percentage points for both Hispanics (15.1) and blacks (11.3). In addition, the online samples rarely yielded accurate estimates of the marginal effects of being Hispanic or black on substantive outcomes, when controlling for other demographics. These results suggest that researchers using online nonprobability samples are at risk of drawing erroneous conclusions about the effects associated with race and ethnicity.
- A representative demographic profile does not predict accuracy. For the most part, a sample’s unweighted demographic profile was not a strong predictor of the accuracy of weighted survey estimates. For example, the two samples with the lowest overall accuracy ranked very highly in terms of how well their unweighted demographics aligned with population benchmarks.4 The implication is that what matters is that the respondents in each demographic category are reflective of their counterparts in the target population. It does not do much good to get the marginal distribution of Hispanics correct if the surveyed Hispanics are systematically different from Hispanics in the larger population.
- One of the online samples consistently performed the best. Sample I consistently outperformed the others including the probability-based ATP, ranking first on nearly all of the dimensions considered.5 This top-performing sample was notable in that it employed a relatively elaborate set of adjustments at both the sample selection and weighting stages. The adjustments involved conditioning on several variables that researchers often study as survey outcomes, such as political ideology, political interest and internet usage. Our impression is that much of sample I’s success stems from the fact that it was designed (before and/or during fielding) to align with the population benchmarks on this broader array of dimensions. Unfortunately, we cannot rigorously test that assertion with the data at hand because we have just one survey from that vendor and the relevant design features were not experimentally manipulated within that survey. While the fact that sample I was conditioned on variables that are often treated as survey outcomes raises important questions, it still appears that the sample I vendor has developed an effective methodology. The results from this study suggest that they produce a more representative, more accurate national survey than the competition within the online nonprobability space.
- Relative to nonprobability samples, results from the ATP are mixed. Pew Research Center’s probability-based panel, the ATP, does not stand out in this study as consistently more accurate than the nonprobability samples, as its overall strong showing across most of the benchmark items is undermined by shortcomings on estimates related to civic engagement. It had the lowest average estimated bias on measures unrelated to civic engagement (4.1 percentage points), but was essentially tied with three other samples as having the largest bias on those types of questions (13.4 points). A likely explanation for this pattern is that the ATP is tilted toward more civically engaged adults as a consequence of being recruited from a 20-minute telephone survey about politics. While the civic engagement bias is concerning, additional analysis indicates that it is not generating large errors on estimates for other domains. When we re-weight the ATP to align with the Current Population Survey (CPS) to eliminate that bias, there is very little impact on other survey estimates, including estimates of voting, party identification, ideology and news consumption.6 In this study the ATP is not intended to represent all probability samples in any meaningful way, but rather provides one point of comparison. It is an open question as to how a one-off telephone random-digit-dial (RDD) survey or some other probability-based survey would stack up in this analysis.
- All of the online samples tell a broadly similar story about Americans’ political attitudes and recreational interests. All of the samples indicate that more U.S. adults consider themselves Democrats than Republicans, though as a group they all tilt more Democratic than dual frame telephone RDD surveys. In addition, all of the samples show that Democrats and Republicans are polarized with respect to their attitudes about the proper scope of government. To be sure, there are some notable differences in certain point estimates – e.g., the share of Republicans who say government is doing too many things better left to businesses and individuals is either 64% or 82%, depending on whether one believes sample F or sample I. The broad contours of Americans’ political atittudes, however, are arguably similar across the samples. By the same token, results from a battery of 11 personal interest items – ranging from gardening to hip-hop music – show that the top-ranking items tend to be the same from one online sample to the next.
This report focuses on the online nonprobability survey market as it currently exists. But much of the current academic and applied research on this subject is focused on how such samples can be improved through modeling. Aside from relatively simple “raking” adjustments, this study did not examine the potential benefits of more elaborate methods for correcting biases.
To address this, additional research reports on online nonprobability sampling are being planned. One will examine a variety of methods of adjustment to determine how well the accuracy and comparability of estimates across nonprobability samples can be improved. The research underway will test different and more complex approaches to weighting (some of which have been employed by researchers in other organizations) and assess the efficacy of these in reducing bias.
A second study will examine the reliability of repeated measurement over time using online nonprobability samples. The ability to track change over time has been one of the key strengths of probability surveys.7
What a ‘probability’ sample does (and does not) mean for data quality
In this report we make a distinction between samples recruited from a design in which nearly everyone in the population has a known, nonzero chance of being selected (“probability-based”) versus samples recruited from advertisements, pop-up solicitations and other approaches in which the chances that a given member of the population is selected are unknown (“nonprobability”). For decades, survey researchers have tended to favor probability samples over nonprobability samples because probability samples, in theory, have very desirable properties such as approximate unbiasedness and quantifiable margins of error that provide a handy measure of precision. For researchers who study trends in attitudes and behaviors over time, the sheer stability of probability-based sampling processes represents an additional crucial property.
While the differences between probability and nonprobability samples may be clear conceptually, the practical reality is more complicated. The root of the complication is nonresponse. If, for example, 90% of the people selected for a probability sample survey decline to respond, the probabilities of selection are still known but the individual probabilities of response are not. In most general population surveys, it is extremely difficult to estimate probabilities of response with a high degree of accuracy. When researchers do not know the probabilities of response, they must rely on weighting to try to correct for any relevant ways in which the sample might be unrepresentative of the population.
Increasingly, researchers are pointing out that when a probability-based survey has a high nonresponse rate, the tools for remediation and the assumptions underpinning the survey estimates are similar if not identical to those used with nonprobability samples. Nonprobability surveys and probability surveys with high nonresponse rates both rely heavily on modeling – whether a raking adjustment, matching procedure, or propensity model – to arrive at what researchers hope are accurate, reliable estimates.