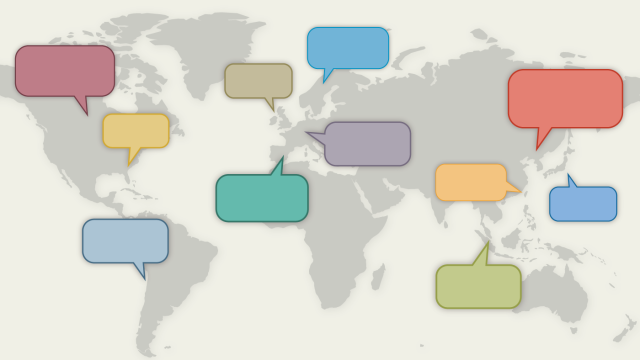
Open-ended survey responses — in which respondents answer poll questions in their own words — are never easy for researchers to analyze. But they can present even bigger challenges when they are written in multiple languages. Few research organizations are likely to have staff members who are fluent and well-versed enough in all of the relevant languages and cultures to analyze such responses with confidence.
Pew Research Center recently tackled this challenge for a cross-national survey in which people around the world described, in their own words, where they find meaning in life. The analysis plan for this project hinged on developing a closed-ended codebook and applying it to nearly 19,000 open-ended responses, drawn from 17 societies and spanning 12 languages.
In this post, we provide more background about this project and look more closely at a key question we faced along the way: whether to use professional translators or Google Translate to help make sense of the many thousands of open-ended responses we received.
Background
At the beginning of this project, we wrestled with three options for translating and coding the more than 11,000 non-English responses we collected about where people find meaning in life.
The first option was to hire a translation firm to translate the responses into English so our researchers could read them and apply a Center-developed codebook to them. On the plus side, this approach would allow researchers to read each verbatim response themselves and have total control over how the codebook would be applied. On the negative side was the huge expense this method would entail. It would be costly not just financially, but also in terms of time spent, since it would require both a translator to read every response as they translated it and a researcher to read each response to code it, potentially extending the length of the project.
A second option was to translate all of the non-English responses using Google Translate. This approach would be free and extremely fast, while again allowing researchers to have total control over how to apply the codebook. However, this approach might have introduced the possibility of error if the Google-translated English text was inaccurate or difficult for researchers to parse — a key concern since the project involved nuanced, highly personal responses about where people find meaning in their lives.
A third option — and the one that we ended up using — was to employ a professional translation firm, but rather than having the translators translate the responses into English, they would simply code the answers in their original language, using the Center’s codebook. This method had a few advantages. First, it would only cost about a quarter as much as having full translations for each verbatim response. Second, it would allow local experts to offer their cultural expertise to ensure that all nuances in the verbatim responses were captured. Third, it would be relatively fast since the translators themselves would do the coding. However, this approach had one large drawback: Researchers at the Center would not be in control of how the codebook was applied and did not necessarily have the language skills needed to verify that it was being applied correctly.
In the end, we mitigated this problem by having the professional translators send us full verbatim translations and their applied codes for a random 10% of open-ended non-English responses from each country and language pairing in our study (e.g., Mandarin in Singapore, Mandarin in Taiwan, Spanish in Spain, Spanish in the United States, etc.). We were then able to clarify any confusion the translators had with the Center’s codebook and verify that the translators and our own researchers had appropriately high levels of intercoder reliability before they began independently coding the responses.
Exploratory analysis
While we were satisfied with the option that we ultimately chose, we still wanted to understand whether Google Translate would be a viable option for potential future research projects of this kind. To do this, we went back and recoded the 10% of non-English responses that we had professionally translated — but this time, we coded versions from Google Translate instead. In the remainder of this post, we’ll explore how our results would have been similar (or different) had we applied our codebook to English translations from Google Translate instead of using professional translators who applied the codebook themselves.
As a benchmark, we’ll compare these two approaches against the decisions our own coders made using the professionally translated versions of the same responses. The two measures presented in this analysis are (1) the intercoder reliability of in-house researchers coding professionally translated responses versus in-house researchers coding responses translated by Google Translate (“In-house researcher reliability using Google Translate” in the table below) and (2) the intercoder reliability of in-house researchers coding professionally translated responses versus professional local researchers coding original-language responses themselves (“Professional translator reliability using native language” in the table below).
To evaluate our intercoder reliability, we used two different versions of Krippendorf’s alpha, a common measure of coding agreement. When looking at each of the 20 codes in our codebook by themselves, we computed alphas traditionally using simple binary flags: whether a coder labeled the response with the code or not. When looking at the codebook overall across all 20 codes, we used a version of Krippendorf’s alpha weighted by Measuring Agreement on Set-Valued Items (MASI) distance. Whereas a traditional Krippendorf’s alpha treats any disagreement on any code as a complete disagreement, weighting by MASI distance provides a small amount of “partial credit” if coders agree on most of the labels in the codebook.
Overall, our in-house coders seemed to code Google-translated responses with the same consistency as professional translators using original-language responses. Across the full non-English sample, the MASI-distance Krippendorf’s alphas for the professional translators and our in-house coding of Google-translated responses were 0.70 and 0.67, respectively. However, while the two methods appeared to be comparable on the whole, our results suggest that Google Translate may perform notably worse in certain languages and in certain contexts.
Results by language
We received roughly 1,000 non-English responses in each language in our survey, with the exception of Malay (under 100) in Singapore. Due to these relatively small sample sizes, we could only get a general sense of intercoder reliability for each of the languages in our sample. Still, the performance of our in-house coders using Google Translate was similar to that of the native-language coders at the professional translation company for most of the languages included in our study.
There were two notable exceptions to this pattern: For Greek and Korean responses, our Google Translate coding was markedly worse than the translators. With Greek responses, coding by professional translators resulted in a Krippendorf’s alpha of 0.76. By contrast, in-house coders using Google-translated responses only reached a score of 0.58. Similarly, the Krippendorf’s alpha for Korean responses coded by professional translators was 0.83, while the same responses coded by in-house coders using Google Translate had a Krippendorf’s alpha of 0.66.
Consider one Greek-language example that produced a notable difference between Center coders using Google Translate and professional translators. The Google-translated response — “No supply matter matter data of the statements we are” — made it difficult for our researchers to assign any of the codes in the codebook. On the other hand, the professional translation — “There is nothing that satisfies me now given the situation we live in” — clearly fell into the “difficulties and challenges” category, according to the rules of our codebook.
Results by code
When considering results by each code individually, the findings were generally similar: Both the in-house coding of Google-translated responses and the coding of nontranslated responses by an outside firm resulted in sufficient agreement with our baseline on most topics. But there were two exceptions: “Don’t know/refused” responses and mentions of service and civic engagement.
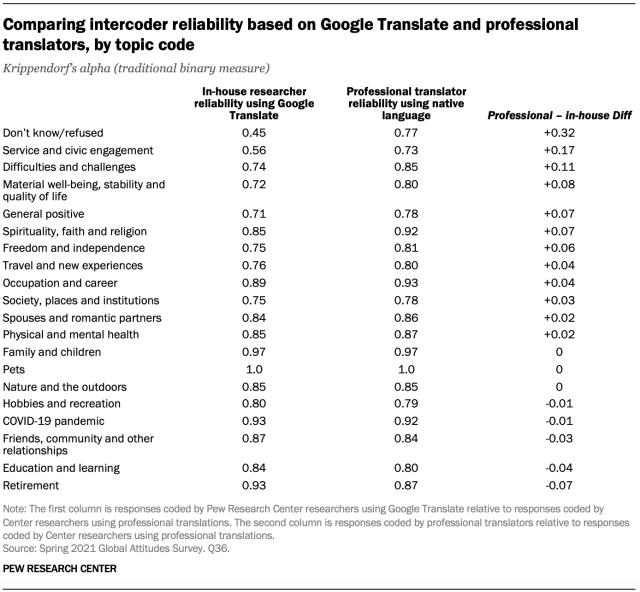
In-house coders using Google-translated responses were considerably less likely to agree with our baseline about when a participant did not answer the question (Krippendorf’s alpha of 0.45). By contrast, agreement by the outside firm reached 0.77. Part of this confusion likely stemmed from the fact that many Google-translated responses said “none” — making it difficult, without sufficient knowledge of the language and context, to clearly parse as people saying they had nothing which gave them meaning in life (which would be coded as “difficulties and challenges”) versus those who simply gave no response (which should have been coded as “don’t know/refused”).
The same was true for mentions of things like service and civic engagement. In-house coders achieved agreement with the baseline of just 0.56 on Google-translated responses, while the outside firm reached an agreement score of 0.73.
Across other codes, there were few differences. Both in-house coders and outside professionals achieved sufficient agreement with the baseline (at or above 0.70) for the remaining categories included in the analysis.
Conclusion
Our findings suggest that Google Translate may provide an adequate substitute for professional translation, but only for some languages and in some contexts.
While we were able to achieve acceptable intercoder reliability in most cases, our coding of Google-translated responses was noticeably worse than the native-language professional translators for Greek and Korean responses. And for a handful of particular topics — response refusals, as well as mentions of service and civic engagement — the Google-translated responses seemed to lack crucial context. In these cases, our own coding of the Google-translated versions of the responses was very different from how we coded the professionally translated versions, while the professional translators who coded the responses in their native language tended to agree with how we coded their translations ourselves.
Of course, there are many considerations in any research project, and the trade-offs related to coding complexity and language must be stacked up against other concerns, including cost and time. Overall, there is no one-size-fits-all answer for all research projects, and Pew Research Center will remain open to various options depending on the particular project at hand.