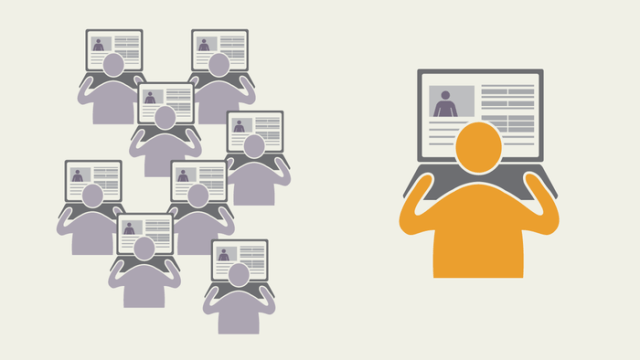
Audiovisual content is everywhere in the digital age, from online news sites to YouTube. Measuring the content and meaning of videos is an important research goal for social scientists, but the tools for analyzing them at scale lag behind new advances in automated text analysis or the analysis of individual images as data. Videos are complex, and reducing them to transcripts or snapshot images might not provide a complete sense of what they convey.
Luckily, researchers already have a tool for measuring meaning in videos: human content coders. Asking trained human coders to watch videos and determine what they convey is a well-established approach, and using interrater reliability statistics helps ensure that human coders are on the same page when making their decisions. But human coding is often slow, expensive and difficult to organize and oversee.
As a result, researchers are increasingly turning to online labor markets such as Amazon’s Mechanical Turk (mTurk) to recruit human coders for content classification tasks that involve text, audio and video. But the question remains: Can online workers with minimal training and oversight do their job as effectively as trained, in-house content coders whose work has been validated with reliability statistics? A new paper says the answer is yes — as long as you combine the decisions of multiple online workers.
In a project I completed with two collaborators at the University of Virginia (Nicholas Winter and Lynn Sanders) before joining Pew Research Center, I compared the quality of content coding done by mTurk workers and several trained undergraduate students. Overall, the online workers performed as well as the trained research assistants when their decisions were grouped together, despite the fact that we never met them in person or provided any direct feedback on their work. At the same time, using online workers had the benefit of forcing us to make our content coding system easily reproducible. Other researchers who want to build on or replicate our work can copy the entire coding interface directly.
The study focused on political advertisements from TV broadcasts. These short videos are a popular subject in political science and have been analyzed by many researchers interested in the effects of political communication more generally.
We asked groups of online workers and in-house content coders to watch political ads and provide information about each one. That information ranged from very cut-and-dried determinations (does the candidate appear in the ad?) to more interpretive assessments (on a scale from very liberal to very conservative, where would you place this candidate based on this ad?).
Below is a screenshot of the video coding interface. Both the mTurk workers and the research assistants completed their tasks using the same interface. However, we organized multiple training sessions for the research assistants in which we rehearsed the coding process and answered any questions.
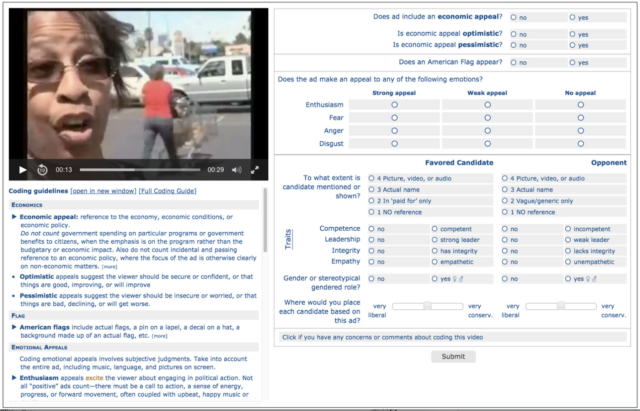
We compared the performance of each group of coders by calculating Krippendorff’s Alpha, a common measure of coder agreement that adjusts for the fact that some attributes of a video appear more or less often across all videos. Higher values of Krippendorff’s Alpha (which is bounded by 0 and 1) mean that the coders agree with each other more often.
For the simple question of whether or not a candidate appears in a given ad, the research assistants reached an alpha value of 0.92, indicating a high level of reliability. The individual mTurk workers got to 0.86 — still relatively high, but not quite as good. However, since content coding is considerably less expensive on mTurk compared with in-house research assistants, we combined the ratings achieved by five separate mTurk coders and compared the result with the combined ratings achieved by five other mTurk coders.
For example, if four out of five workers said a candidate appeared in an ad, we rounded that decision to a single “yes” for the question. Then we compared that “yes” with the rounded decision of another batch of five coders who saw the same ad. In other words, if the majority of workers in the second group agreed that the candidate appeared, that would also be treated as a “yes,” and both groups would agree. (If the two groups of five coders reached different conclusions, we treated it as a disagreement.)
Using these grouped ratings, we found that reliability increased beyond that of the research assistants, all the way to 0.97. In other words, the rounded decisions of five mTurk workers, compared with other combined batches of five workers, were even more reliable than those made by the trained research assistants.
Other coding items were more complicated. For example, we also wanted to measure the ideological signals candidates send in ads. These signals are complex, and might be communicated both verbally (“I am a strong liberal/conservative”) or visually (video of the candidate appearing with well-known liberal or conservative leaders).
To measure these signals, we asked coders to place the candidates who were favored and/or opposed in each ad on a liberal to conservative scale with 101 potential options, where 0 is very liberal and 100 is very conservative. These kinds of measurements are valuable for social scientists. By measuring ideology at the ad level, for example, researchers can study how campaigns signal liberal or conservative identities over time and across different parts of their constituencies.
Overall, the research assistants reached a reliability coefficient of 0.66 when rating candidates’ ideology, which was lower than for the more basic question of whether the candidate appears in the ad or not. Individual mTurk workers did much worse, with a Krippendorff’s Alpha of just 0.43. But again, combining multiple mTurkers made a difference. Comparing randomly selected groups of five mTurk coders with each other increased the reliability score to 0.68.
The full set of results for each of the coding items appears in the image below. The groups of five randomly selected mTurk coders are referred to as “metacoders” and their answers are combined and compared with other sets of five mTurkers.

Using multiple mTurk coders instead of just one improved reliability across the board. And the combined decisions of these online workers were as reliable or better than those made by individual research assistants.
Using online workers is a major time saver for researchers, though the costs in this project were about the same. We spent about $0.60 including fees to code the same ad five times on Mechanical Turk. Individual research assistants, paid at an hourly rate, averaged about one ad for $0.56. But the mTurk workers were much faster overall and worked all hours. It would take about one week to code 2,000 ads five times each using the online workers. It would require a very large team of research assistants to match that output.
What’s more, using the online workers allowed us to fully document and capture every aspect of the coder experience, including instructions. That level of detail is important if content coding projects are meant to be reproducible or replicable.
Overall, this research shows that crowdsourcing for content classification is viable. Researchers can measure both simple and more interpretive aspects of videos using online workers, and they can attain similar levels of cross-coder reliability as research assistants would provide. As the volume of video content continues to increase, researchers should conduct additional comparisons to confirm that these results apply across domains and research questions.
Replication data for the paper is available here.